TensorFlow is an end-to-end open source platform for machine learning. TensorFlow is a rich system for managing all aspects of a machine learning system; however, this class focuses on using a particular TensorFlow API to develop and train machine learning models. See the TensorFlow documentation for complete details on the broader TensorFlow system.
TensorFlow APIs are arranged hierarchically, with the high-level APIs built on the low-level APIs. Machine learning researchers use the low-level APIs to create and explore new machine learning algorithms. In this class, you will use a high-level API named tf.keras to define and train machine learning models and to make predictions. tf.keras is the TensorFlow variant of the open-source Keras API.
The following figure shows the hierarchy of TensorFlow toolkits:
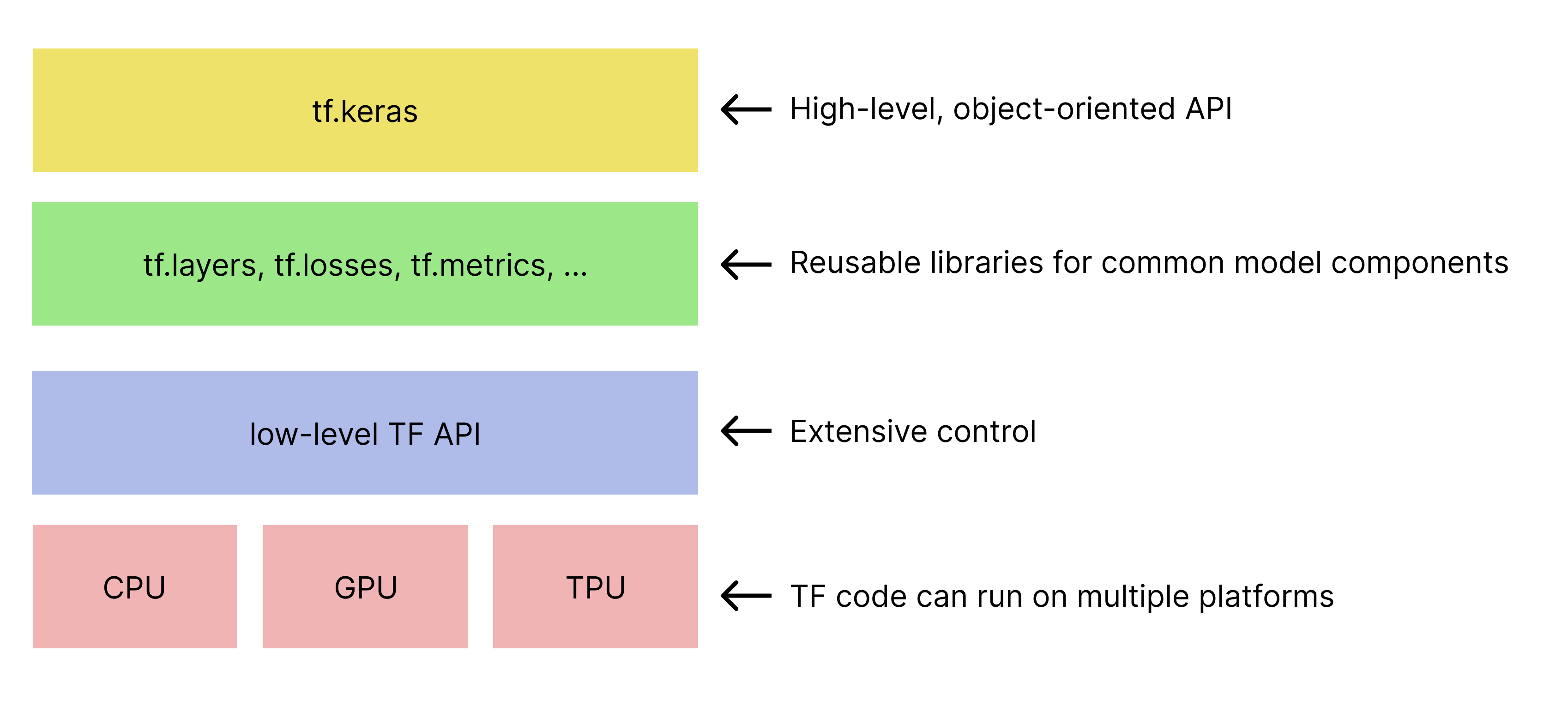
Figure 1. TensorFlow toolkit hierarchy.